Research Contributions:
RF Clouds Intelligence
Research in cyberspace applications benefiting from the RF cloud of the wireless devices at CWINS began with motion and gesture detection and authentication and security and more recently moved into interference measurement and modeling for intelligent spectrum management.
2021
2020
BESTNEST Research Center
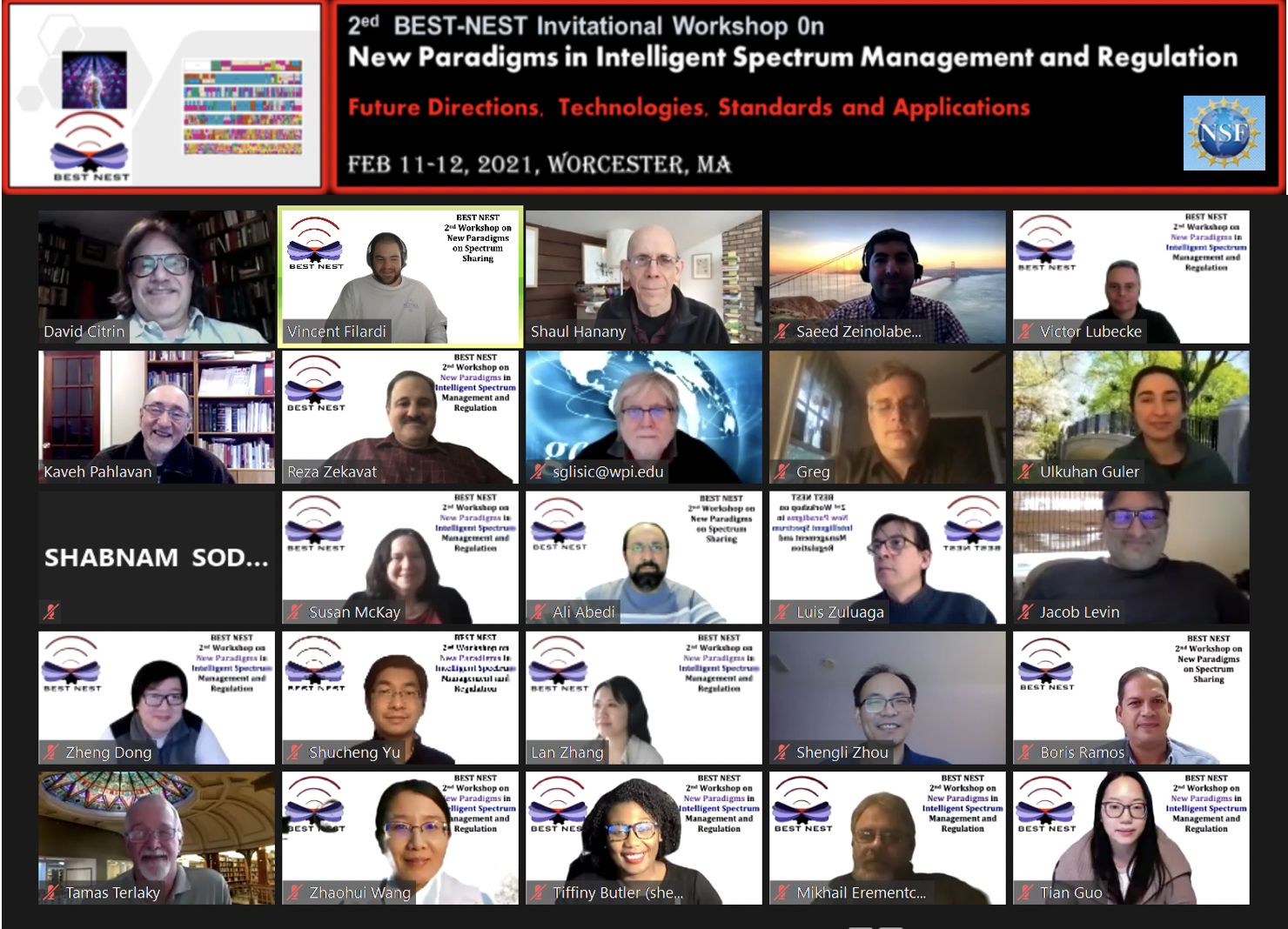
Major Projects
ThuMouse
- Ziheng Li
- Zhenyuan Lei
- An Yan
- Erin Solovey
- Kaveh Pahlavan
IndexPen
- Kaveh Pahlavan
- Emanuel Agu
- Rick Brown
- Sergey Makarov
- Robert Kineck
- John Orr
Using the IEEE 802.11 WLAN access points, PCMCIA WLAN cards, EKAHAU positioning software, and laptops acquired from the CNS support and our PROPSIM real-time channel simulator acquired from a DoD grant we have completed a novel laboratory testbed for performance evaluation of the indoor positioning systems operating based on RSS. This is the first laboratory testbed that is developed for performance monitoring of indoor positioning systems. The novel methodology adopted in this experiment uses our existing Ray-tracing software to identify location of the training points and measure the performance of the positioning software (in this case EKAHAU engine). The WLAN access points and a laptop with PCMCIA cards as well as the Ray-tracing software are connected to the real-time channel simulator that simulates the channel behavior between location of the access points and the mobile, specified in the Ray-tracing GUI. This innovative setup allows low cost repeatable performance analysis of indoor positioning systems. Our first publication in this field describes the architecture of the testbed and the preliminary results of performance evaluation that relates the density of the training points to the accuracy of the measurements.